Case Study
Optimisation Algorithms in a Regional Vacuum Sewer Utility
Alexandrina Council transformed a regional brownfield vacuum sewer from break-fix to real time optimisation in under 2 months using Data Science.
The Challenge
From a vacuum sewer brownfield headache to fast transformation using algorithms.
In 2021, Alexandrina Council’s Water Department set an aspiration to become the smartest regional wastewater utility in Australia built on the back of an IoT-to-AI strategy.
However, this ambition was challenged almost immediately, when they took on the regional responsibility to acquire a brownfield vacuum sewer from a private property development on Hindmarsh Island. The challenges included ageing infrastructure, incomplete documentation and a complete lack of network process monitoring capability. Operationally flooded lines, rainfall ingress, valve malfunctions and call outs were, anecdotally, common occurrences. The challenge was how to transform internal capability, knowledge and ensure safe and efficient operation in the fastest possible time.
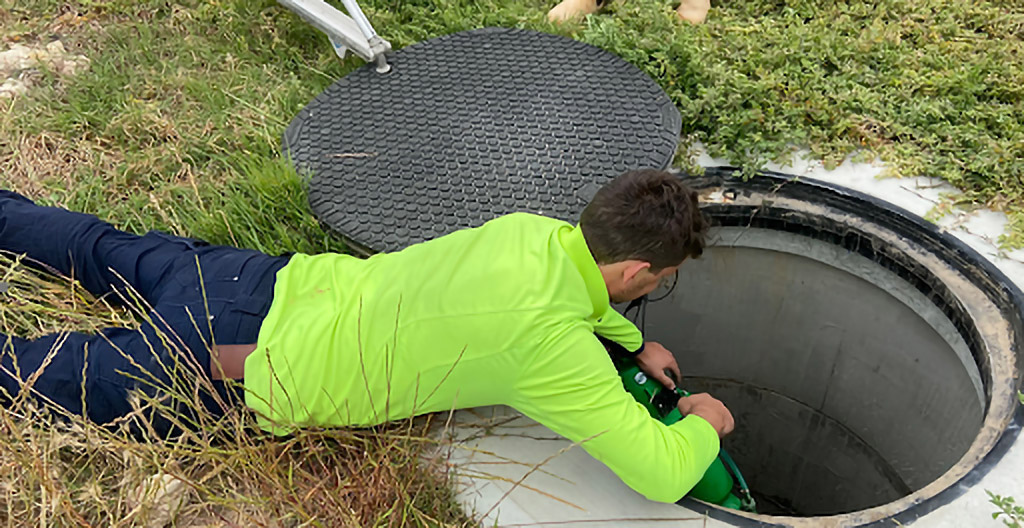
Sensors: Alexandrina rolled out valve and pressure sensors measuring number of valve activations, valve average opening time and line pressure.
Algorithm-First Approach
Co-Design, iterations and data-first.
Rather than invest more field operators or engineering assessments at the Hindmarsh Island vacuum sewer, Alexandrina used data to inform them of the path forward. Moving quickly, they rolled out an IoT sensing network over the vacuum system and then engaged SpiralData, experts in algorithms for water asset optimisation. SpiralData worked with Alexandrina using co-design and agile principles to quickly move through: feasibility study, problem backlog, prioritised algorithm sprints, build-test-deploy, proof-of-concept then pilot, and finally, solution support and maintenance.
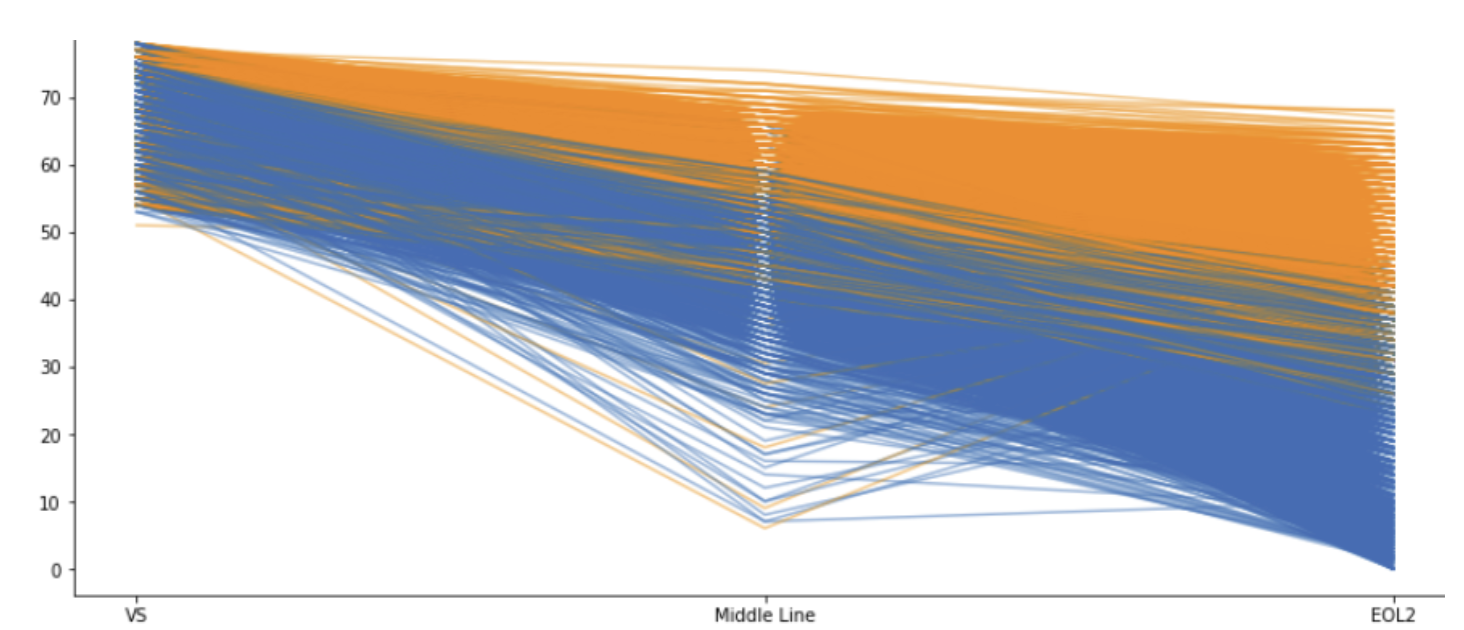
End of line pressure: Chart shows linear pattern of pressure from the vacuum station (VS) to the middle of the line (middle line) and to the end of the line (EOL.) By understanding through a linear regression we were able to estimate pressure anywhere along the line as an input into the optimisation model.
The Platform
Enabling data science and machine learning teams to deliver business value
For rapid deployment of a data lake and AI workbench, SpiralData leveraged our infrastructure-as-code AI platform-as-a-service (AI PaaS.) This meant less time standing up infrastructure and more time for the Data Scientists to work with their favourite tooling to solve real-world water utility problems. Hosted on AWS this includes microservices such as AWS SageMaker, Anaconda, Jupyter, VSCode, BitBucket, Dask, Datashader, Bokeh, Plotly-express, AWS CloudFormation.
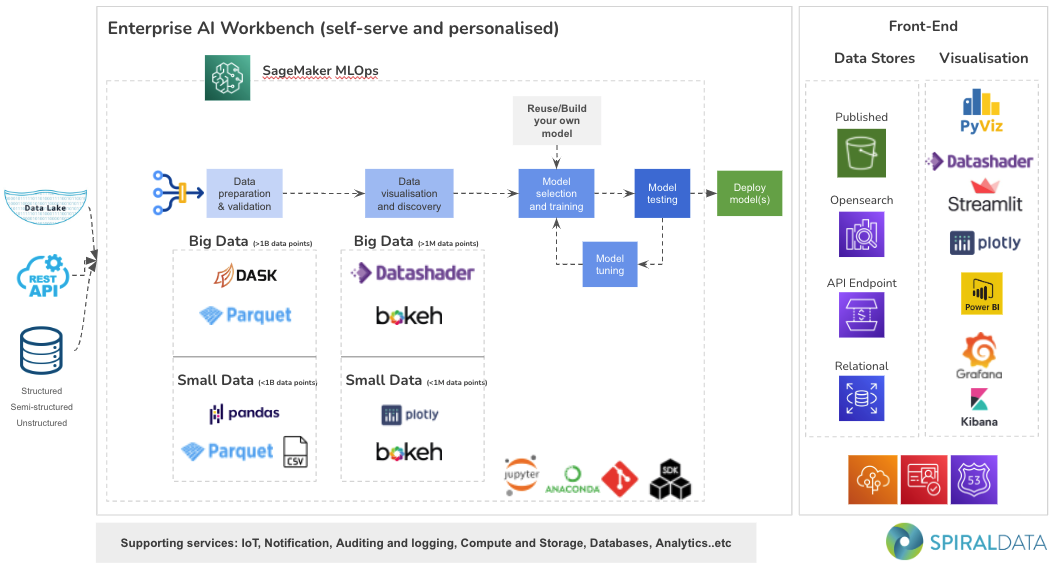
Our AI PaaS reference architecture: Our unified platform leverages the oversight and control of SageMaker on AWS but enables practitioners to self-manage with unlimited personalised tooling options. Experiments can be tracked and shared easily, enabling reproducible data science that is secure and scales.
The Results
1) Algorithms solve rainfall ingress triage for operations
To-date, rainfall ingress has been identified in the vacuum sewer at 100% accuracy using the algorithm. Over time it is anticipated that reduced rainfall ingress will result in:
Video: showing rainfall ingress at a specific pit identified by the correlation algorithm. Rainfall ingress (not septic) can be clearly seen.
2) Algorithms optimise valves reducing flooded lines and improving efficiency
Lower impacts from higher influent demands on the end-of-line pressures resulted in:
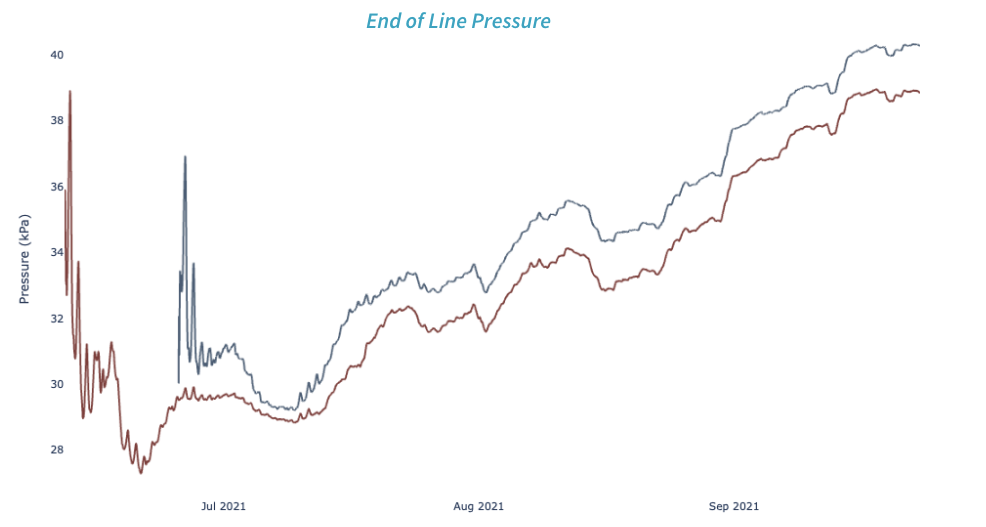
End of Line Pressure Trend for Vacuum Sewer. The 30 day moving average of end-of-line pressure within the vacuum sewer utility at Alexandrina Council. A global supplier recommends a minimum of 30 kPa target. A consistent trend upwards indicates overall system performance improving.
Learnings for Water Utilities
1
What worked:
- Agile process of algorithm development (interaction + intensity of effort)
- An assigned water professional as the Product Owner
- Site visits, co-design and remote build with a multidisciplinary team
2
Lessons learned:
- Key resources need support to commit to dedicated projects
- Time box project meetings unless exploration activities
- Ground-truth algorithms, don’t assume the output is incorrect
3
Wishlist:
- Extend platform to all of the vacuum sewer
- Algorithms for leak detection, optimising vacuum pumps and air injectors
- Rollout to the rest of Alexandrina’s wastewater gravity network